
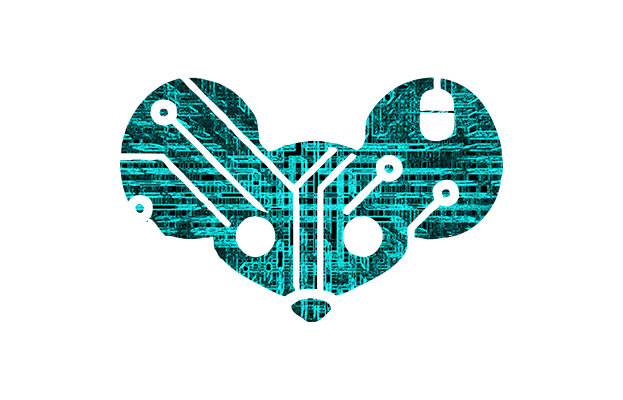
Yup, they just stopped pretending. For years they thought they had to keep up a friendly facade for us peasants, but Trump showed them that it’s no longer needed.
Yup, they just stopped pretending. For years they thought they had to keep up a friendly facade for us peasants, but Trump showed them that it’s no longer needed.
“we were using ChatGPT to design our server architecture and…” (OpenAI PR tomorrow, probably…)
exactly! “I was energized to meet with the team in X and discuss our sales figures” or “congrats, company Y, for disrupting the market of foot creams” is the best use of AI.
I’m not sure how you would even be able to tell if that type of content is AI-generated or just plain old copy-pasted from one of a thousand similar posts
if you want to listen to 35 minutes of annoying music, press 1. If you want to get insulted personally by an operator stay on the line
I don’t think Musk would disagree with that definition and I bet he even likes it.
The key word here is “significant”. That’s the part that clearly matters to him, based on his actions. I don’t care about the man and I don’t think he’s a genius, but he does not look stupid or delusional either.
Musk spreads disinformation very deliberately for the purpose of being significant. Just as his chatbot says.
I think I’m with him on this one. Replacing all the people on social with AI agents would give us back so much free time! And we could even restart socializing for real.
Go on Zuckerberg, give us a Facebook made only of AI agents creating fake pictures of inexistent gatherings and posting them, so other AIs can recommend them and million of other AIs can comment on them!
You are an unsung hero, Zuckerberg, but one day they’ll understand and thank you
on the other hand, when Putin’s done killing off most of their own present and future workforce in a senseless war and completely tanking his own economy, that might be the equivalent of like $3
Socials and the Internet in general would be a much better place if people stopped believing and blindly resharing everything they read, AI-generated or not.
I’m not sure we, as a society, are ready to trust ML models to do things that might affect lives. This is true for self-driving cars and I expect it to be even more true for medicine. In particular, we can’t accept ML failures, even when they get to a point where they are statistically less likely than human errors.
I don’t know if this is currently true or not, so please don’t shoot me for this specific example, but IF we were to have reliable stats that everything else being equal, self-driving cars cause less accidents than humans, a machine error will always be weird and alien and harder for us to justify than a human one.
“He was drinking too much because his partner left him”, “she was suffering from a health condition and had an episode while driving”… we have the illusion that we understand humans and (to an extent) that this understanding helps us predict who we can trust not to drive us to our death or not to misdiagnose some STI and have our genitals wither. But machines? Even if they were 20% more reliable than humans, how would we know which ones we can trust?
Most things to do with Green Energy. Don’t get me wrong, I think solar panels or wind turbines are great. I just think that most of the reported figures are technically correct but chosen to give a misleadingly positive impression of the gains.
Relevant smbc: https://www.smbc-comics.com/comic/capacity
I think they don’t matter with outrage, because outrage explodes in ways that are hard to predict. I mean, I can see the problem with the ad now that it has been pointed out to me. After reading about it repeatedly, I now find it bad and ridiculous and what were they thinking? But at a first look, as a test audience I would have probably rated it as “meh, ok”.
It is about fragility, like others said, but It is also about uniqueness, in the sense of “oh, so you think you’re soo special!”
ah I get what you’re saying., thanks! “Good” means that what the machine outputs should be statistically similar (based on comparing billions of parameters) to the provided training data, so if the training data gradually gains more examples of e.g. noses being attached to the wrong side of the head, the model also grows more likely to generate similar output.
AKA “shit, looks like now we need to re-hire some of those engineers”
TBH those same colleagues were probably just copy/pasting code from the first google result or stackoverflow answer, so arguably AI did make them more productive at what they do
I only have a limited and basic understanding of Machine Learning, but doesn’t training models basically work like: “you, machine, spit out several versions of stuff and I, programmer, give you a way of evaluating how ‘good’ they are, so over time you ‘learn’ to generate better stuff”? Theoretically giving a newer model the output of a previous one should improve on the result, if the new model has a way of evaluating “improved”.
If I feed a ML model with pictures of eldritch beings and tell them that “this is what a human face looks like” I don’t think it’s surprising that quality deteriorates. What am I missing?
deleted by creator
About 20 new cases of gender violence arrive every day, each requiring investigation. Providing police protection for every victim would be impossible given staff sizes and budgets.
I think machine-learning is not the key part, the quote above is. All these 20 people a day come to the police for protection, a very small minority of them might be just paranoid, but I’m sure that most of them had some bad shit done to them by their partner already and (in an ideal world) would all deserve some protection. The algorithm’s “success” in defined in the article as reducing probability of repeat attacks, especially the ones eventually leading to death.
The police are trying to focus on the ones who are deemed to be the most at risk. A well-trained algorithm can help reduce the risk vs the judgement of the possibly overworked or inexperienced human handling the complaint? I’ll take that. But people are going to die anyway. Just, hopefully, a bit less of them and I don’t think it’s fair to say that it’s the machine’s fault when they do.
I have to admit It was a solid idea, though. Dick pics should be one of the best training sets you can find on the internet and you can assume that the most prolific senders are the ones with the lowest chance of having an STI (or any real-life sexual activity).
Goldman Sachs, quote from the article:
Generative AI can indeed do impressive things from a technical standpoint, but not enough revenue has been generated so far to offset the enormous costs. Like for other technologies, It might just take time (remember how many billions Amazon burned before turning into a cash-generating machine? And Uber has also just started turning some profit) + a great deal of enshittification once more people and companies are dependent. Or it might just be a bubble.
As humans we’re not great at predicting these things including of course me. My personal prediction? A few companies will make money, especially the ones that start selling AI as a service at increasingly high costs, many others will fail and both AI enthusiasts and detractors will claim they were right all along.