
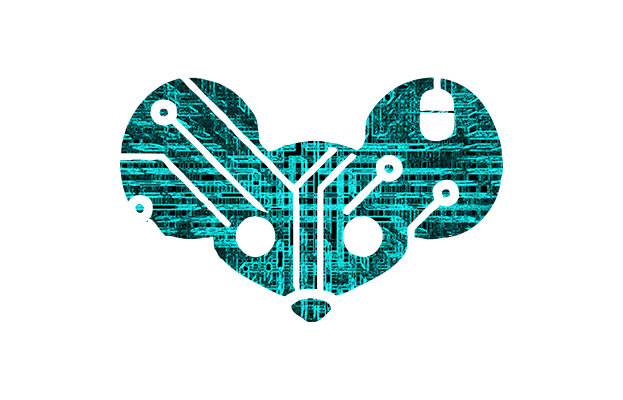
1·
7 months agoYeah, the problem is how to sanitise effectively. You’ve gotta be able to find a way to automatically strip out “bad” things from your training data (via an “oracle”). But if you already had that oracle, you could just slap it on your final product (e.g. Search) and make all the “bad” things disappear before they hit the user (via some sort of filter).
Another great example (from DeepMind) is AlphaFold. Because there’s relatively little amounts of data on protein structures (only 175k in the PDB), you can’t really build a model that requires millions or billions of structures. Coupled with the fact that getting the structure of a new protein in the lab is really hard, and that most proteins are highly synonymous (you share about 60% of your genes with a banana).
So the researchers generated a bunch of “plausible yet never seen in nature” protein structures (that their model thought were high quality) and used them for training.
Granted, even though AlphaFold has made incredible progress, it still hasn’t been able to show any biological breakthroughs (e.g. 80% accuracy is much better than the 60% accuracy we were at 10 years ago, but still not nearly where we really need to be).
Image models, on the other hand, are quite sophisticated, and many of them can “beat” humans or look “more natural” than an actual photograph. Trying to eek the final 0.01% out of a 99.9% accurate model is when the model collapse happens–the model starts to learn from the “nearly accurate to the human eye but containing unseen flaws” images.