
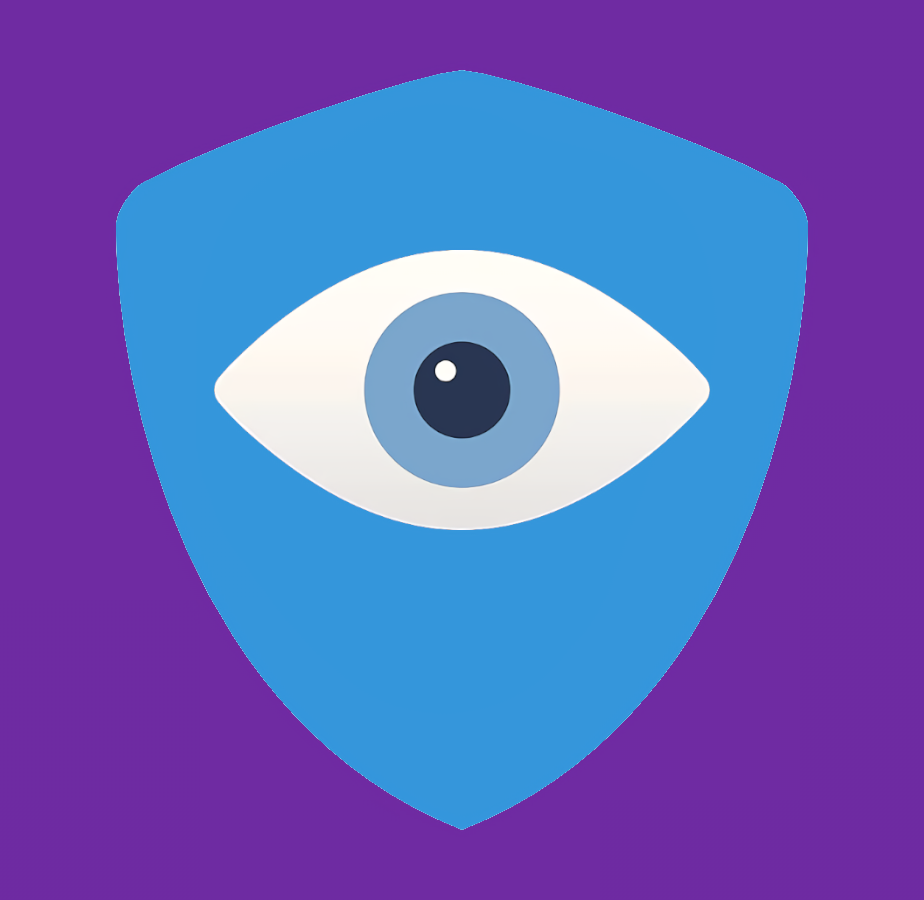
Right – all privacy-positive methods to employ, but not helpful for fingerprinting. In fact, some things can make you more susceptible to fingerprinting because they make you more unique (like using a custom OS). It’s all about your browser and what it chooses to send with HTTP requests, how it responds to queries for you device/browser specs (via Javacript). Your OS, system architecture, hardware details, browser type and plugins, etc combine to make a very unique profile tied to your device. It’s especially nefarious because all those bits are cross-referenced over all accounts and devices to make a global profile on you. Even if you’ve never used Facebook, you probably have a shadow profile. If you’ve ever logged into the same service or website account on your de-Googled GrapheneOS device as another machine that does have Google services tracking, then your new device is likely already tied to your identity.
Try this with different browsers – it tests the uniqueness of your device.
Ban me too, please? I’d prefer to not stumble across whatever you’re hosting.